MSP IR Automation: Orchestrating Incident Response with SOAR
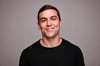
If you’ve ever tried to run incident response at an MSP without automation, you know the feeling. It's like trying to conduct a symphony with a kazoo, a spatula, and three people who showed up late and...
Read more